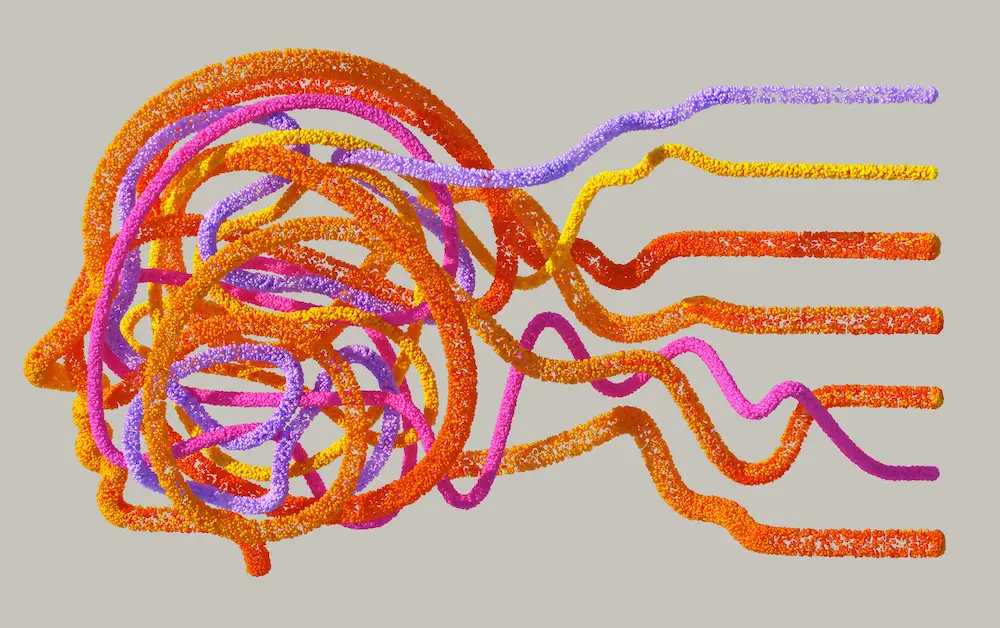
Algorithmic Fairness and Addressing Bias
Algorithms, despite being powered by advanced technology, are creations of humans and are inherently influenced by the culture, perspective, and biases—both implicit and explicit—of their creators. Human thoughts, shaped by cultural perceptions, attitudes, and stereotypes, are mirrored in algorithms designed to replicate human behavior. Consequently, if human behavior is biased, so too can be the algorithms they create. Algorithmic bias refers to a systematic error that leads to unfair, inaccurate, or unethical outcomes, excluding certain groups from opportunities.
For fintech companies, it is crucial to ensure that individuals responsible for developing AI programs undergo training on applicable fair lending and antidiscrimination laws. This training should empower them to identify discriminatory outcomes and address them responsibly. Analyzing data inputs can help identify potential selection bias or the incorporation of systemic bias, minimizing the risk of algorithms generating discriminatory outputs. Responsible use of algorithms, according to CGAP, involves understanding the variables considered in credit scoring models and how they affect individuals’ scores. For example, using data that identifies applicants as immigrants without proper consideration may perpetuate financial exclusion.
Researchers have suggested using separate algorithms to classify different groups of people instead of applying the same measures universally. Legislation has also been proposed to recommend companies to publish technical details or limited datasets for review and testing to detect potential discrimination. Other approaches include creating independent bodies to review proposed datasets and establishing best practices guidelines for developing nondiscriminatory AI systems. Unchecked, unregulated AI can amplify bias, highlighting the need for awareness and accountability in AI to prevent unfavorable use.
Several companies, organizations, and government bodies are taking positive steps to mitigate biases in AI. Microsoft’s Fairness, Accountability, Transparency, and Ethics in AI (FATE) project aims to provide AI-powered services without discrimination. Efforts like Women in Machine Learning (WiML) and Black in AI Workshop focus on building diverse talent in AI. Microsoft Research and Boston University have developed a method to identify and offset biases in algorithm results, creating a less biased dataset. Google’s GlassBox initiative aims to make machine-learning algorithms more understandable without compromising output quality.
Women’s World Banking, in partnership with data.org, is working on including gender awareness in credit scoring algorithms. They have developed a tool to estimate bias in credit models and emphasize the importance of debiasing data for developers and implementing checks for institutional management.
Regulatory technology (regtech) has made significant progress in managing algorithmic bias risks by developing smart algorithms. Financial services companies are expected to leverage third-party regtech algorithms for testing and monitoring smart algorithms in credit transactions. Regulators are also adopting regtech solutions, fostering better coordination among regulators and unprecedented opportunities for collaboration between regulators and institutions.
The Algorithmic Fairness Act of 2020 was introduced in Congress to increase fairness and transparency in algorithmic eligibility determinations. The Consumer Financial Protection Bureau (CFPB) aims to address digital redlining and algorithmic bias in its fair lending supervision and enforcement efforts. Ongoing efforts include identifying emerging risks, developing policy responses, and expanding examination of AI, machine learning, and automated valuation models in lending.
Mitigating algorithmic biases is an ongoing process, requiring transparency among developers and mechanisms for understanding and monitoring biases. In developing financial markets with limited regulations, investors and supervisors should be well-informed about the variables used in algorithms and how excluded groups are treated.